Quantum computing is transforming the field of protein structure prediction, a key step in drug discovery. It uses quantum systems’ unique properties like superposition and entanglement. This allows for faster and more accurate molecular modeling and protein folding simulations.
Despite its challenges, predicting protein structures is crucial. Classical computing’s limitations make it hard. Quantum machine learning offers new solutions, opening doors for drug design innovation.
Accurate protein structure prediction is vital for understanding diseases and developing targeted treatments. Quantum computing could help identify new drug targets and improve lead compounds. This could speed up the drug discovery process, leading to better treatments for patients.
In this article, we’ll explore the challenges of protein structure prediction. We’ll see how quantum computing can change this field. We’ll also discuss current uses and future possibilities at the intersection of quantum technology and drug discovery.
The Challenges of Protein Structure Prediction in Drug Design
Protein structure prediction is vital in drug design, as knowing a protein’s 3D structure is key to creating targeted treatments. Yet, predicting protein structures accurately is a daunting task. The protein folding problem, which aims to find a protein’s native 3D structure from its amino acid sequence, is particularly challenging. This is due to the vast number of possible conformations proteins can take.
Proteins move through complex energy landscapes, making it hard to pinpoint the most stable and biologically relevant structure. The number of possible structures grows exponentially with protein size. Experimental methods like X-ray crystallography and NMR spectroscopy help, but they’re limited by the size and complexity of proteins they can study.
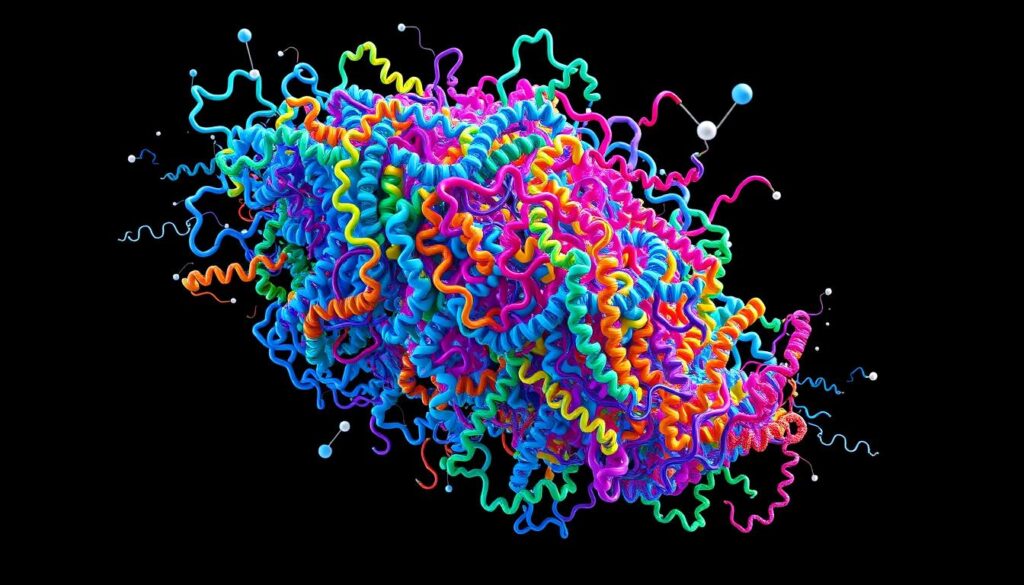
Computational methods offer a way to complement experimental techniques, aiming to predict protein structures from sequences. However, these methods face their own challenges due to the vast size and complexity of proteins. The search space for possible conformations is enormous, requiring significant computational power to evaluate each structure’s stability and accuracy.
To tackle these challenges, researchers employ various strategies. These include:
- Advanced algorithms and heuristics to efficiently explore the conformational space
- Using experimental data to guide and constrain the prediction process
- Applying machine learning to learn from known protein structures
- Utilizing high-performance computing to meet the demands of structure prediction
Despite these strategies, protein structure prediction methods still need improvement. Developing more accurate and efficient computational tools is essential. This is crucial for speeding up drug design and discovery, which heavily depend on understanding protein-drug interactions at a structural level.
How Quantum Computing Can Revolutionize Protein Structure Prediction
Quantum computing is poised to transform protein structure prediction, a critical step in drug development. Quantum computers can tackle complex calculations and simulations at speeds classical computers can’t match. This is due to quantum speedup, a unique property of quantum systems.
Protein structure prediction faces a major hurdle: the number of possible conformations grows exponentially with protein size. Quantum optimization algorithms, like QAOA and VQE, offer a solution. They efficiently navigate the vast conformational space to find the most stable, energetically favorable structures.
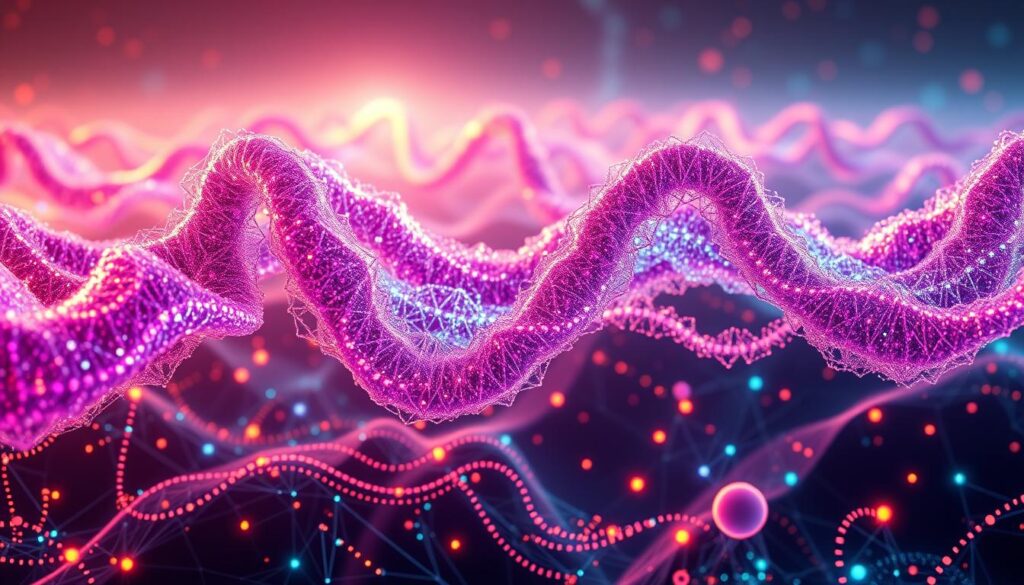
Quantum sampling techniques, including quantum annealing and adiabatic quantum computation, also play a key role. These methods allow for more efficient exploration of the energy landscape. This leads to the discovery of low-energy conformations that classical methods might miss.
Moreover, quantum computers’ inherent parallelism enables the simultaneous evaluation of multiple protein conformations. This significantly cuts down the time needed for protein structure prediction. The result is a faster drug discovery process, with researchers quickly identifying effective drug candidates.
The combination of quantum algorithms and sampling techniques with existing tools for protein structure prediction could overcome classical computing’s limitations. By leveraging quantum computing, researchers can tackle bigger, more complex proteins. This will improve prediction accuracy and speed up the development of new, effective drugs.
Improving Protein Structure Prediction for Drug Design Using Quantum Computing
Quantum computing is set to transform protein structure prediction, especially in drug design. It uses quantum-enhanced molecular modeling for more accurate and efficient simulations of drug-protein interactions. This method offers deeper insights into protein dynamics and interactions with drug candidates.
Quantum machine learning also shows great promise for enhancing protein structure prediction models. Training quantum algorithms on large datasets of known protein structures leads to more precise models. These models can predict protein 3D structures with unmatched accuracy, speeding up drug discovery by identifying new targets and optimizing docking simulations.
Quantum computing’s role in drug discovery could drastically cut down development time and costs. It allows for exploring vast chemical spaces to find promising drug candidates. This streamlines the early drug discovery stages, focusing on the most viable compounds.
Furthermore, quantum computing improves the accuracy and efficiency of protein-ligand docking simulations. These simulations are key to understanding drug interactions with target proteins. By including quantum effects, researchers get more realistic and reliable predictions of binding affinities and conformational changes. This leads to the creation of more effective and selective drugs.
In conclusion, the integration of quantum computing in protein structure prediction and drug design is highly promising. It leverages quantum-enhanced molecular modeling, quantum machine learning, and quantum-assisted drug discovery. This approach can accelerate the development of new therapeutics, enhancing patient outcomes.
Current Quantum Computing Applications in Protein Structure Prediction
Quantum computing experiments have shown promising results in protein structure prediction. Researchers are using advanced quantum hardware and software to tackle the complex challenge of determining protein folding patterns. They aim to revolutionize drug design and development with quantum algorithms implementation.
One notable approach is quantum annealing for protein folding simulations. D-Wave Systems, a leading company in quantum computing, has collaborated with researchers. They applied their quantum annealing technology to this problem. This technique explores vast conformational spaces, enabling the identification of low-energy protein structures. It has shown potential in accurately predicting the structures of small proteins.
Another promising avenue is the quantum variational algorithm. It combines classical optimization methods with quantum circuits. Researchers at Google AI Quantum have demonstrated its successful implementation for protein folding simulations. By iteratively optimizing a parameterized quantum circuit, they generated accurate protein structures. This hybrid approach leverages the strengths of both classical and quantum computing.
Quantum simulation has also emerged as a powerful tool in protein structure prediction. Teams at IBM Quantum have conducted experiments using their quantum hardware to simulate small proteins. By mapping the protein system onto a quantum circuit, they studied the dynamics and interactions of amino acids. These quantum simulations provide valuable insights into the folding process and guide the design of novel therapeutic proteins.
While these quantum computing applications are still in their early stages, they have already demonstrated significant potential. As quantum hardware and software advance, we can expect more accurate and efficient protein structure prediction methods. These developments will accelerate drug discovery efforts and enable the design of targeted therapies for various diseases.
Future Prospects and Challenges of Quantum Computing in Protein Structure Prediction
Quantum computing has the potential to transform protein structure prediction. However, several challenges must be overcome to fully exploit its capabilities. The main issue is quantum computing scalability, as current hardware is limited by the number of qubits. To accurately simulate complex proteins, quantum computers need a significant increase in capacity.
Improving quantum error correction techniques is also crucial. This is necessary to reduce the inherent noise and errors in quantum systems. Such advancements will ensure reliable and precise simulations of protein folding.
Developing novel quantum algorithms for protein folding is another key area of focus. Researchers need to design efficient algorithms that utilize quantum computers’ unique features, like superposition and entanglement. These algorithms should be optimized for quantum hardware advancements. This will unlock new insights into protein folding dynamics and speed up drug discovery.
Interdisciplinary collaboration is vital for the success of quantum computing in protein structure prediction. Experts from quantum computing, computational biology, and drug discovery must work together. Their combined knowledge will help develop practical solutions for protein folding simulations. This collaboration will be essential as quantum computing evolves, driving innovation and breakthroughs in the field.