The transition to renewable energy sources is underway, with wind power gaining traction as a viable alternative to fossil fuels. However, the challenge of optimizing wind farm layouts for peak energy production remains daunting. Traditional methods often fall short, resulting in less than optimal energy outputs. Quantum computing offers a groundbreaking solution, transforming the way we approach wind turbine placement and computational modeling.
Quantum algorithms hold the key to enhancing energy efficiency in wind farm design. They utilize quantum systems’ unique properties to explore an immense number of layouts swiftly. This could result in the creation of highly efficient wind farm configurations. Such configurations would maximize renewable energy production while reducing costs and environmental impact.
This article will explore the realm of quantum computing and its role in optimizing wind farm layouts. We will examine the shortcomings of traditional optimization techniques and highlight quantum algorithms’ potential to overcome these hurdles. Through real-world examples and groundbreaking research, we will illustrate quantum computing’s transformative impact on the wind energy sector. It promises a more sustainable future.
Introduction to Wind Farm Layout Optimization
Wind farm layout optimization is crucial in wind farm design. It significantly impacts the energy output and efficiency. The main aim is to boost power generation while cutting down construction and maintenance costs. This involves a detailed site assessment, taking into account wind speed, direction, and the wake effect.
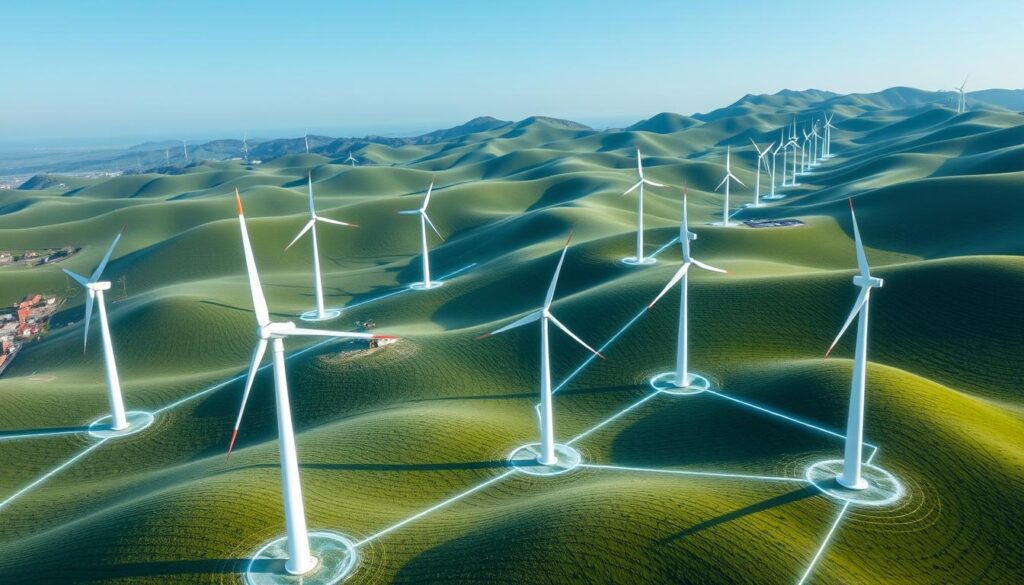
The wake effect happens when turbines are too close, reducing wind speed and increasing turbulence. This can greatly lower the farm’s energy output. Therefore, optimizing turbine spacing is key to reducing this effect. By analyzing wind patterns, developers can find the best spacing for turbines to enhance energy production.
Optimizing wind farm layout involves several critical factors:
- Wind speed and direction
- Terrain and topography
- Turbine specifications and performance
- Environmental and social constraints
- Electrical infrastructure and grid connection
By carefully weighing these factors and using advanced optimization methods, developers can design layouts that fully exploit wind resources. This approach minimizes environmental impact and costs. As the need for clean energy grows, the role of wind farm layout optimization in wind energy success and sustainability becomes even more vital.
Traditional Methods for Wind Farm Layout Optimization
Wind farm layout optimization has traditionally relied on various mathematical modeling and computational methods. These aim to maximize energy production while minimizing costs. Computational fluid dynamics (CFD) simulations are a key technique, analyzing wind flow and turbine interactions for efficient layouts.
Genetic algorithms, inspired by natural selection, are another popular method. They start with an initial population of layouts and iteratively improve them. The best layouts are selected based on energy output and cost, creating the next generation.
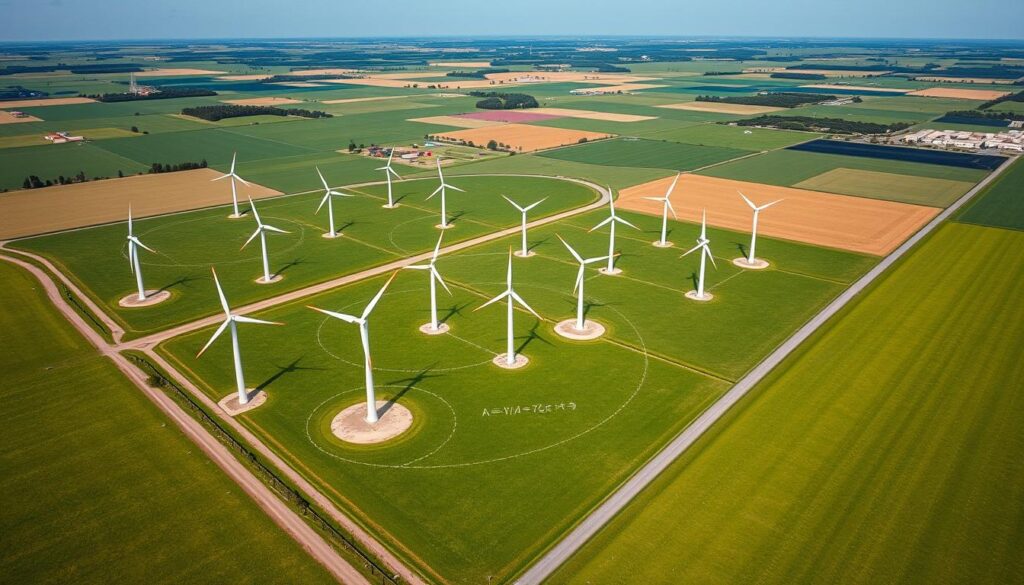
Heuristic methods, like the greedy algorithm and simulated annealing, are also used. These methods use simplified rules for efficient search, often sacrificing accuracy. While successful in improving designs, they face challenges with scalability and complex real-world constraints.
As wind farms grow, traditional methods’ limitations become clear. Simulating and optimizing large farms is expensive and time-consuming. These methods also struggle with real-world variables and constraints, such as terrain and environmental regulations.
Quantum Computing: A Promising Solution
Quantum computing is seen as a breakthrough for wind farm layout design, overcoming traditional optimization methods’ limitations. It uses quantum mechanics to perform complex calculations, solving problems faster than classical computers. Quantum algorithms, like quantum annealing and quantum optimization, show great promise in optimizing wind farm layouts.
At the core of quantum computing is the qubit, the quantum information unit. Unlike classical bits, qubits can be in multiple states at once, known as superposition. This allows quantum computers to explore many possibilities in parallel, solving complex problems more efficiently.
Quantum annealing is a quantum algorithm well-suited for optimization problems. It encodes the problem in qubits and slowly evolves towards the optimal solution. This process explores many potential solutions, finding the global optimum faster than classical methods.
Quantum optimization is another promising approach. It uses quantum algorithms to solve complex problems. Formulating wind farm layout optimization as a QUBO problem, it can be efficiently solved. This method could significantly reduce the time needed to find the optimal layout, leading to more efficient and cost-effective wind farms.
The use of quantum computing in wind farm layout optimization is still in its infancy. However, the potential benefits are substantial. Quantum algorithms could lead to wind farms with higher energy production, lower costs, and less environmental impact. As quantum computing advances, we can expect to see more applications in renewable energy.
Optimizing wind farm layouts for maximum energy production quantum computing
Quantum computing is transforming wind farm layout optimization, offering innovative solutions for boosting energy yield. Researchers are crafting quantum-inspired algorithms and hybrid quantum-classical methods. These innovations blend quantum computing’s power with classical optimization techniques. The goal is to improve computational efficiency and scalability, tackling complex wind farm scenarios.
Quantum computing excels in efficiently exploring vast solution spaces. Quantum algorithms use superposition and entanglement to evaluate multiple layouts simultaneously. This identifies the most energy-efficient configurations, crucial for large-scale wind farms where possibilities grow exponentially.
Hybrid quantum-classical approaches leverage both quantum and classical computing strengths. Quantum algorithms efficiently explore the solution space, while classical techniques refine the layouts. This synergy effectively manages complex scenarios, considering terrain, wind patterns, and turbine interactions.
Quantum annealing is another method being explored for wind farm optimization. It’s a quantum computing technique well-suited for optimization problems. By applying quantum annealing to wind farm layouts, it finds the global optimum more efficiently than classical methods. This has shown great promise in improving layout quality and efficiency.
Quantum computing’s application in wind farm layout optimization promises significant energy yield improvements. By using quantum algorithms and hybrid approaches, developers can find the most optimal layouts. This maximizes energy production while considering various constraints, enhancing project efficiency and cost-effectiveness.
As quantum computing evolves, its impact on wind farm optimization will expand. Advances in quantum algorithms and hardware will boost efficiency and scalability. This will enable the optimization of larger, more complex wind farms, driving renewable energy adoption and supporting a sustainable future.
Case Studies and Real-World Applications
Quantum computing’s advancement is unlocking its potential in optimizing wind farm layouts. This is happening through collaborations and pilot projects with energy companies. EDF Renewables and Equinor are teaming up with D-Wave Systems and IBM. They aim to apply quantum algorithms to real-world wind farm design challenges.
A notable case study involves optimizing an offshore wind farm layout with a D-Wave Systems quantum annealer. Researchers formulated the problem as a quadratic unconstrained binary optimization (QUBO) problem. This allowed them to use the quantum annealer’s power to find optimal solutions more efficiently than classical methods. The outcome showed an increase in energy production and a decrease in wake losses. This highlights quantum computing’s potential to enhance the performance of large-scale offshore wind farms.
Another successful pilot project is the collaboration between Vattenfall and Cambridge Quantum Computing (CQC). They applied CQC’s quantum algorithms to Vattenfall’s wind farm data. This led to identifying optimal layouts that maximized energy production while reducing costs. This case study showcases quantum computing’s value in solving complex optimization problems in wind energy.
As more energy companies see the potential of quantum computing for wind farm layout optimization, we can expect more commercial deployment. The success of these early pilot projects and case studies is paving the way. It’s leading to the integration of quantum computing into wind energy’s design and planning processes. This will result in more efficient and cost-effective wind farms in the future.