The fields of quantum computing and materials science are rapidly evolving. A groundbreaking approach is emerging, with immense potential for revolutionizing superconducting materials discovery. Quantum algorithms and computational methods are accelerating the process. They unlock insights into superconductivity’s complex physics.
This article explores the intersection of quantum physics, materials science, and advanced computational techniques. We’ll see how quantum-assisted design is transforming superconductor research. It enables scientists to predict and optimize new superconducting materials with high accuracy and efficiency.
Join us as we delve into the challenges and opportunities of this emerging field. Discover how quantum-assisted materials discovery is set to revolutionize advanced superconductor development. Witness the power of quantum computing as it reshapes our understanding of superconductivity and opens new frontiers in materials science.
Introduction to Quantum-Assisted Materials Design
Quantum-assisted materials design is a groundbreaking field that uses quantum computing to speed up the creation of new materials. It employs quantum algorithms and simulation to predict and enhance materials properties with unmatched precision. This innovation could revolutionize sectors like energy, electronics, healthcare, and aerospace.
At its heart, quantum-assisted materials design tackles complex calculations that classical computers can’t handle. Quantum computers, by exploiting quantum phenomena, can explore vast spaces in parallel. This enables the quick identification of potential materials, cutting down on the time and expense of traditional methods.
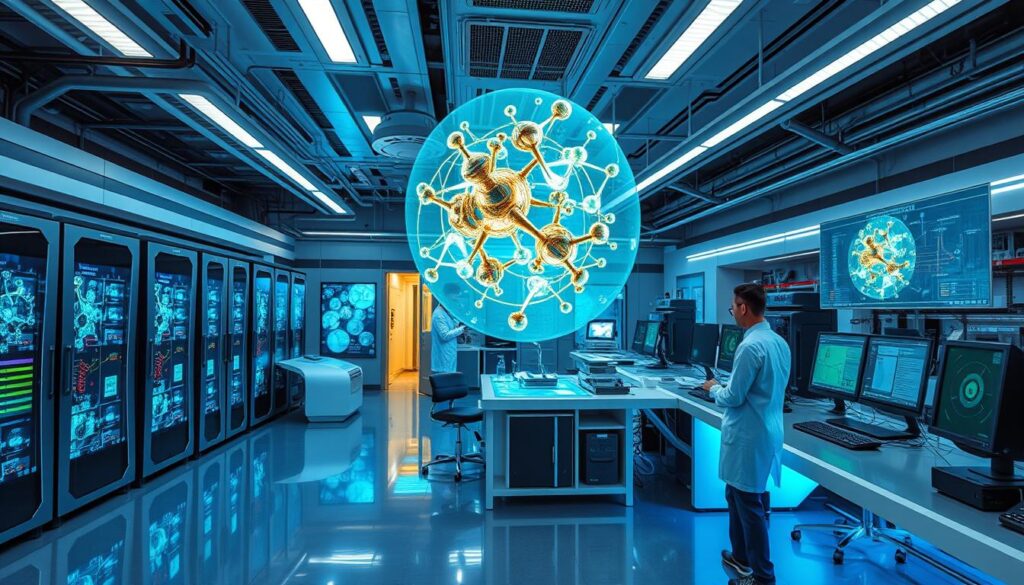
This approach excels in predicting materials properties before they’re made. By simulating quantum-level interactions, researchers understand materials’ electronic, magnetic, and optical properties. This predictive capability allows for the design of materials with specific functions, like superconductors or efficient catalysts.
Quantum algorithms in materials design go beyond just predicting properties. They help find stable crystal structures and uncover hidden patterns in materials data. These advanced methods, combined with quantum computers’ power, efficiently explore the vast chemical space.
For quantum-assisted materials design to reach its full potential, collaboration among scientists is key. Developing software that connects quantum algorithms to simulations is vital. Also, integrating these methods with experimental techniques will be necessary to validate predictions.
In conclusion, quantum-assisted materials design marks a significant shift in material discovery and development. It leverages quantum computing and simulation to accelerate the process, predict properties accurately, and design materials with specific functions. As quantum technology advances, its impact on various industries will expand, ushering in a new era of materials innovation.
Challenges in Superconductor Discovery and Development
The quest for new superconductors is a complex journey, blending materials science, quantum mechanics, and computational methods. The main hurdle is navigating the vast chemical space to find promising candidates. With countless element combinations and crystal structures, traditional methods are slow and costly.
Optimizing the critical temperature of superconductors is another significant challenge. The critical temperature is the threshold below which a material exhibits superconductivity. Achieving high critical temperatures is essential for practical use. Yet, predicting and controlling this temperature is complex, requiring deep physics knowledge and understanding of material properties.
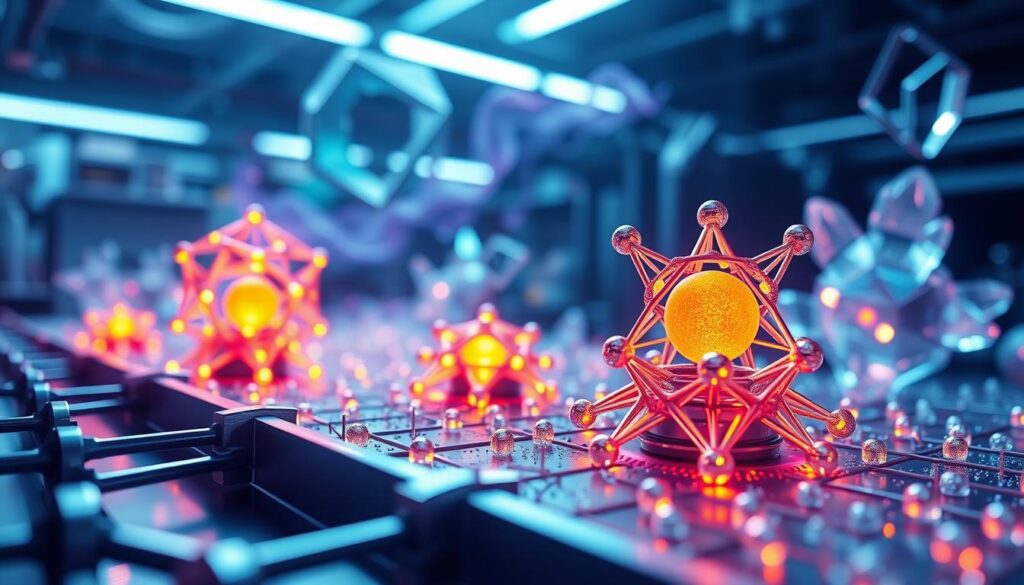
Ensuring materials stability is crucial for superconductor development. Superconductors must withstand various conditions like high magnetic fields, mechanical stress, and thermal fluctuations. Their long-term stability is vital for reliable use in real-world applications.
To overcome these hurdles, researchers are embracing advanced computational methods and quantum-assisted design. Quantum computing and machine learning algorithms speed up the discovery process, identifying promising candidates more efficiently. Quantum-assisted design enables rapid screening of vast chemical spaces, predicting material properties, and optimizing critical parameters like the critical temperature.
Quantum-assisted design plays a pivotal role in superconductor discovery, focusing on:
- High-throughput screening of materials databases
- Quantum simulations of superconducting mechanisms
- Machine learning models for property prediction and optimization
- Inverse design approaches for targeted superconductor discovery
By merging quantum computing with materials science, researchers are poised to accelerate superconductor discovery and development. Quantum-assisted design could unlock new superconductivity frontiers, leading to materials with higher critical temperatures, better stability, and enhanced performance for diverse applications.
Quantum Computing and Its Role in Materials Science
Quantum computing is transforming materials science with its potential to revolutionize it. It uses quantum mechanics to perform complex calculations, surpassing classical computers. This breakthrough in efficiency opens new paths for discovering and optimizing materials, solving problems previously unsolvable.
Simulating complex quantum systems is a key application of quantum computing in materials science. Quantum algorithms like VQE and QAOA efficiently model electron behavior in molecules and materials. They accurately predict material properties, guiding the creation of new materials with specific characteristics.
Quantum computing is also advancing in optimizing materials properties. Algorithms like quantum annealing and quantum adiabatic algorithm efficiently search vast parameter spaces. They find optimal materials compositions and structures faster than classical methods, speeding up the discovery of superior materials.
Combining quantum computing with machine learning is another powerful approach in materials science. Quantum machine learning algorithms analyze large datasets from simulations and experiments. They uncover patterns and correlations, aiding in the design of new materials. This synergy between quantum computing and machine learning accelerates materials discovery and predictive modeling.
Despite its vast potential, quantum computing in materials science faces challenges. Developing robust and scalable quantum hardware is essential. Integrating quantum computing with existing infrastructure and techniques requires significant software development and standardization efforts. However, the rapid advancement in quantum technology and growing interest from materials science indicate a bright future for quantum-assisted materials design.
Quantum-Assisted Design of New Superconductors
Quantum-assisted superconductor design is transforming the field of novel superconducting materials. It uses quantum computing to predict material properties with high accuracy. This method combines advanced algorithms with quantum processors, allowing scientists to explore vast chemical spaces. It helps identify promising candidates for high-temperature superconductivity.
Quantum-assisted materials design excels in handling complex quantum mechanical calculations. Traditional methods struggle with these calculations due to their exponential scaling. Quantum algorithms, however, use quantum superposition and entanglement to efficiently explore vast parameter spaces. This leads to the identification of the most promising materials for further study.
Recent breakthroughs in quantum-assisted superconductor design have led to the discovery of several novel superconducting compounds. For instance, quantum-based techniques predicted high-temperature superconductivity in hydrogen-rich materials under extreme pressures. These predictions were later confirmed through experimental studies, showcasing the power of quantum-assisted design.
As quantum computing advances, the potential for quantum-assisted superconductor design expands. Larger and more powerful quantum processors will enable researchers to tackle more complex materials systems. This will accelerate the discovery of new superconductors and provide deeper insights into superconductivity.
The successful application of quantum-assisted design in superconductor discovery has significant implications. It could transform industries like energy, medicine, and quantum computing. By harnessing quantum computing in materials design, we are making strides towards realizing the full potential of these revolutionary materials.
Computational Methods for Superconductor Simulation
Computational methods are crucial for designing new superconductors. They combine quantum algorithms with classical techniques. This allows for precise and efficient simulation of superconducting materials.
The variational quantum eigensolver (VQE) is a key quantum algorithm. It uses a hybrid method, blending quantum circuits with classical optimization. This approach helps approximate the ground state energy and wavefunction of superconductors. Such information is vital for understanding their electronic properties and identifying superconducting phases.
The quantum approximate optimization algorithm (QAOA) is another powerful tool. It excels in solving optimization problems, like finding the optimal atomic configuration in superconductors. QAOA encodes optimization problems into quantum circuits. It then iteratively applies quantum gates to efficiently search for the best materials.
Classical methods, like density functional theory (DFT), are also essential. DFT calculates a material’s electronic structure from first principles. It solves the many-body Schrödinger equation, describing electron behavior. Combining DFT with machine learning enhances simulation accuracy and efficiency.
Machine learning algorithms, including artificial neural networks and support vector machines, are trained on vast datasets. They identify patterns in superconducting materials. These models predict new material properties, guiding the search for novel superconductors. Integrating machine learning with quantum algorithms and DFT speeds up material discovery and optimization.
The quantum-assisted design of superconductors combines computational methods. This includes quantum algorithms, density functional theory, and machine learning. By using each method’s strengths, researchers gain deep insights into superconductivity. They can also accelerate the development of materials with specific properties.
Experimental Validation of Quantum-Designed Superconductors
Quantum-assisted design has become a key tool for predicting new superconducting materials. Yet, experimental validation is essential to confirm these predictions and assess their practicality. This process includes materials synthesis, characterization techniques, and superconductivity measurements. It verifies the performance of quantum-designed superconductors.
Materials synthesis is the initial step. It involves creating the predicted materials through various methods like solid-state synthesis or chemical vapor deposition. The goal is to achieve the exact composition and crystal structure while maintaining purity. However, maintaining precise composition and avoiding impurities is a significant challenge.
After synthesis, advanced characterization techniques are used to study the materials’ properties. These include:
- X-ray diffraction (XRD) and neutron diffraction to determine the crystal structure and lattice parameters
- Scanning tunneling microscopy (STM) to investigate the local electronic structure and superconducting gap
- Angle-resolved photoemission spectroscopy (ARPES) to map the electronic band structure and Fermi surface
- Transport measurements, such as resistivity and critical current density, to evaluate the superconducting performance
Superconductivity measurements are the final test. They involve cooling the materials to very low temperatures and applying magnetic fields. This determines the critical temperature (Tc) and critical magnetic field (Hc) for superconductivity. Researchers also study how the materials respond to external stimuli like pressure or doping to enhance their properties.
The validation of quantum-designed superconductors is a joint effort between theorists and experimentalists. The exchange between computational predictions and experimental results refines design models. This collaboration aims to unlock superconductivity’s full potential for applications in energy, electronics, and quantum technologies.
Future Prospects and Challenges in Quantum-Assisted Materials Design
Quantum-assisted materials design is on the cusp of significant advancements. Improving the scalability of quantum hardware is a major focus. This will enable the simulation of more complex materials systems. Collaboration between quantum physicists and computer scientists is essential for developing new quantum architectures and error correction schemes.
Moreover, the development of sophisticated quantum algorithms is crucial. These algorithms will be necessary for tackling the increasing complexity of materials design problems. This will be a key factor in the field’s future success.
The creation and maintenance of comprehensive materials databases are also critical. These databases will be invaluable for training quantum machine learning models and validating simulation results. Researchers must establish standardized data formats and metadata schemas. This will ensure the databases’ interoperability and usefulness across different research groups and institutions.
Interdisciplinary collaboration is vital for driving progress in quantum-assisted materials design. Materials scientists, quantum physicists, and computer scientists must work together. They need to bridge the gaps between their fields and develop a common language for addressing challenges.
This collaborative approach will be essential for translating theoretical advancements into practical applications. It will be crucial across various industries, from renewable energy to advanced electronics. As the field matures, establishing clear roadmaps and priorities for research and development is crucial. This will ensure that the most promising avenues are pursued and the benefits of quantum-assisted materials design are realized efficiently.