Table of Contents
One of the key areas that snags the attention of quantum computing (QC) firms is quantum machine learning (QML). In the simplest form, QML is the concept and process of developing QC systems that have the potential to boost the performance of machine learning (ML).
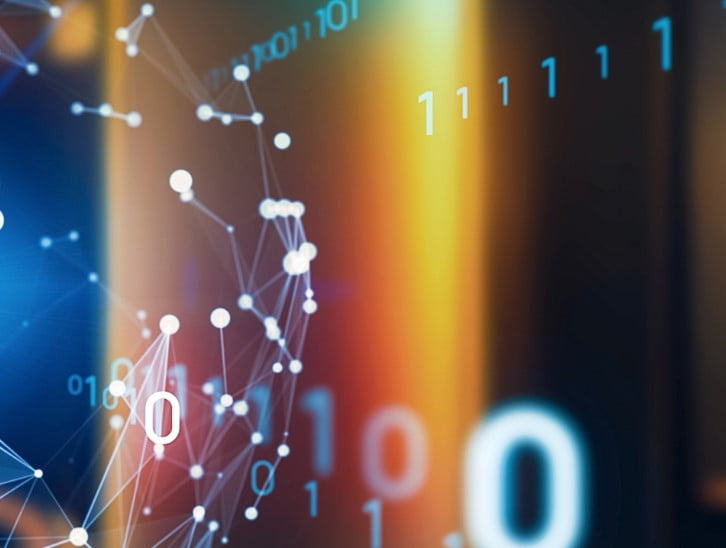
To achieve this, developers integrate quantum programs within the ML programs. QML is most common in areas where users execute conventional data on QC systems. It employs qubits and relevant operations to enhance processing speed, data storage, and data retrieval. Because the QC systems use compatible algorithms, they can analyse their states instead of traditional data.
You can also think of QML as a field of study that investigates the interaction of concepts from QC systems with ML systems or the application of quantum advantage to enhance the performance, efficiency and scalability of trainable networks and programs. For example, because ML techniques entail training neural systems to learn and exhibit specific qualities, it takes time to train them. However, introducing QC systems to the practice could significantly reduce the amount of time computer scientists need to reach that goal.
Quantum Machine Learning Applications
At this point, you will be wondering whether QML is still another classroom concept or has some real-world applications. Indeed, your concern is legitimate, given that today’s QC systems are still grappling with noise, unstable qubits, etc.
To give you a straightforward answer to the question, here’s the catch: some companies are taking bold steps to take QML from the research lab to the real world. Therefore, we will review some of those companies in this article in a jiffy. But then, QML proponents believe the world will use the emerging technology in the following aspects:
- Using reinforced learning to optimise policies for autonomous systems, such as self-driving cars and unmanned aerial vehicles
- Generating more realistic data for Variational Autoencoders (VAEs) and Generative Adversarial Networks (GANs) for effective data augmentation
- Enhancing optimisation-solving algorithms, which have widespread applications in the industry
- Accelerating classification tasks for large datasets, chiefly in image and speech recognition
- Deepening our understanding of the nanoparticles
- Improving neural network training by leveraging interference. This saves training time by reducing the number of iterations needed to obtain the optimal parameters
- Strengthening today’s computer security by combining with it blockchain technology
- Improving our understanding of human anatomy and other related concepts
- Facilitating molecular modelling of drugs and solving chemistry problems for new drug discovery
- Analysing features and anomalies for effective financial risk mitigation and fraud detection
- Using molecular and atomic mapping to enhance the production of novel materials
With the daily spring-up of new ideas and innovations, QML applications could go beyond those areas. As regards industry-specific applications, there are strong indications that users will apply QML to power generation, manufacturing, retail, government services, finance and insurance, aerospace and space, environment and weather, customer service, and social media.
3 Real-World QML Application
Having come thus far, let us review three organisations using the QML technology.
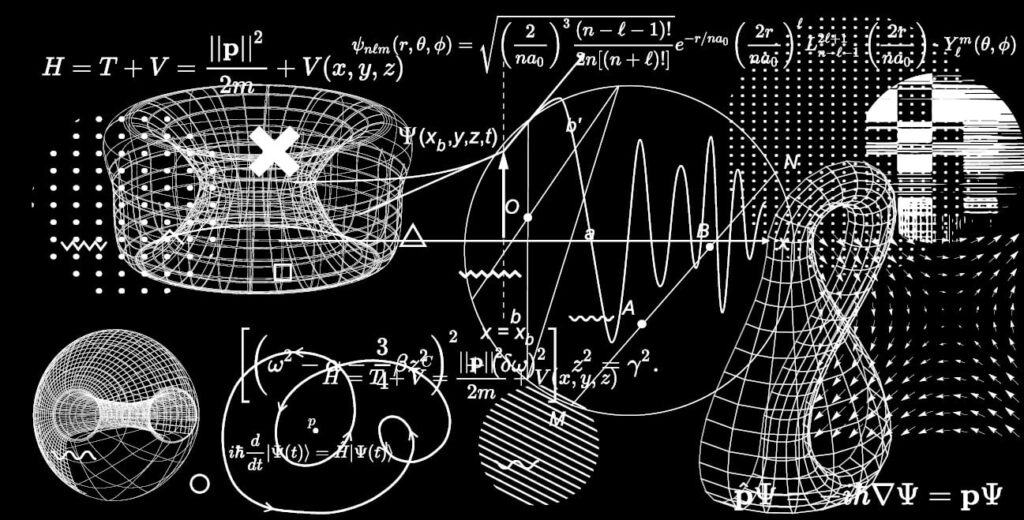
Menten AI
When it comes to the QC technology, Menten AI has shown its deep-rooted belief in harnessing its enormous potential. Consequently, Menten AI is working hard to design peptide and protein therapeutics using QC technology.
The early-stage drug discovery company uses QML to deepen today’s computational methods and make it possible to develop new protein therapeutics from scratch and carve a niche for itself in the pharma industry.
In clear terms, the American pharma company designs protein drugs and enzymes using the QML technology. Given the capacity of QC technology to leapfrog conventional computing, Menten AI can build previously intractable proteins. As a result, the team created the foremost protein-built algorithm for today’s (and future) QC systems.
To make the world better, the company worked on three new proteins and a peptide. The primary objective of the project is to prevent the spread of viruses like the COVID-19 pandemic.
Because the Menten AI team understands the significant role of QML in their industry, they partnered with Xanadu’s open-source library PennyLane to leverage quantum simulations and QML capabilities to enhance their protein-based drugs.
With the recent collaboration that took place in January 2022, Menten AI is now set to bring to bear protein design, ML techniques and the QC technologies. It is worth noting that the business relationship between the drug development company and the Canadian QC company will see the former deploy QML (from PennyLane) to facilitate molecule optimisation and simulation.
The use of QML will also reduce the cost of research and development in the pharma industry and improve time to patients’ hands. On the other hand, Xanadu’s PennyLane integrates scientific computing and ML libraries with the simulators and NISQ devices .
Today, Menten AI’s platform can achieve nM potency directly from in silico design as well as with desirable in vitro and in vivo properties. In pharmacology, potency is the measure of a drug’s biological activity and medical scientists express it in terms of the dose needed to produce the desired effect.
JPMorgan Chase
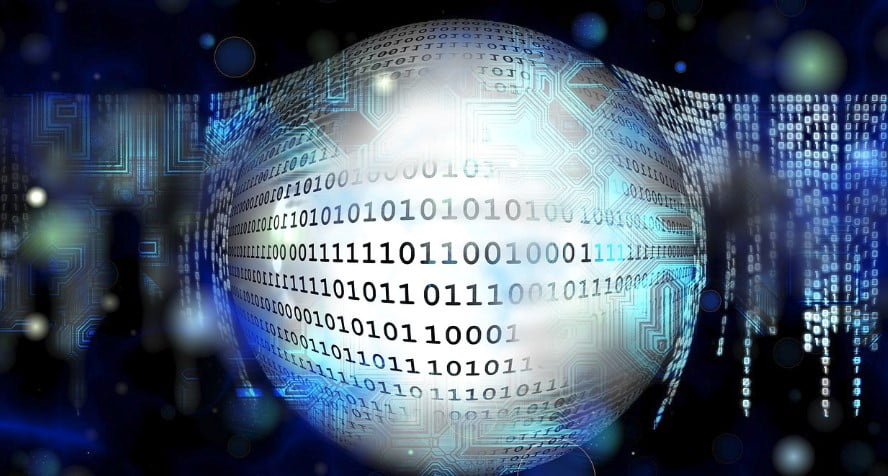
As you are aware, JPMorgan is a force to reckon with in the American financial services industry. In March 2023, the leading financial institution disclosed that it planned to enhance its risk mitigation strategies using QML technology.
To make this happen, JPMorgan partnered with American quantum-computing-as-a-service company QC Ware to examine the critical questions bordered on risk minimisation for portfolio-utilised data-driven models. The study sought to ascertain if they could improve today’s deep hedging frameworks using quantum deep learning.
Then, they used reinforcement learning to examine whether they could define a new framework for deep hedging. At the end of the extensive study, they disclosed that financial services providers could improve deep hedging on conventional frameworks using models based on quantum deep learning to achieve more efficient training.
Delving further into the details of the study, the team carried out the research on Quantinuum’s H1-1, a 12-qubit QC system launched in June 2022. With this development, financial risk management firms can learn more about expectations and distribution of returns, use quantum-actor-critic reinforcement learning models to offer improved performance, and participate in effective policy training.
Interestingly, the strategy could improve deep hedging in both conventional and quantum environments because it leverages QML techniques to enhance time accuracy and trainability of high-performance GPU hardware.
By embracing QML to enhance its risk management services, JPMorgan Chase is solidifying its already robust position in its market and using future-ready programs to maintain that competitive edge in the future. Another way to look at it is that the financial services giant has deployed QML technology to deliver more value to its vast network of investors and rocket its revenue.
It is also interesting that JPMorgan chose QC Ware, a quantum and traditional computing software and services firm that teams up with future-focused enterprises to explore QC capabilities and achieve industry-wide disruptions.
Kuano
In September 2023, Kuano hit the headlines of British media houses for closing a £1.8 million seed funding round. For a brief intro, Kuano is a drug discovery company that uses QML models to design medicines. The pharma company planned to use the funds to facilitate further validation of its quantum simulation platform for the design of more effective drug-candidates-targeting enzymes. It will also help the company further its strategic partnerships.
To gain insight into what Kuano does, you should grasp the concept behind its approach to drug discovery. Dysfunctional enzymes, as you already know, cause many human diseases. In short, they are often the primary targets during drug design.
Today, scientists observe enzymes in the rest state, not when they are fully functioning. However, once they are resting, they appear similar to others.
Therefore, while in their resting state, using new drugs to target defective enzymes often affects others. That, in turn, impacts drug safety and efficacy. To this end, Kuano’s quantum simulation platform (also known as a quantum lens) helps scientists view, observe and model enzymes when they are fully functioning, making it possible to design more potent drugs.
With Kuano’s QML technology, users can easily predict the most appropriate structures for targeting enzymes. The outcome is more potent drugs with little or no side effects. Thankfully, Kuano’s platform has already earned validation in three different disease areas, including bowel cancer and lymphoma.
With funds coming in from funding rounds, the Kuano team is dotting the I’s and crossing its T’s to take the project from the lab to market. Since its establishment in 2020 in the United Kingdom, Kuano has spearheaded exhaustive research that leverages QML techniques to enable faster development of superior drug candidates.
Takeaway
Although many companies are trying out the application of QML in their fields, the organisations above have implemented certain initiatives to change their narratives.
Over the years, QML has been an idea used among physicists and computer scientists in the classrooms and research labs, but the three companies we reviewed in this article are making efforts to take it to the real world. Admittedly, those projects are in their early stages, so the full implementation will take shape in a few years from now.
Furthermore, computer scientists find it challenging that numerous conventional ML algorithms don’t naturally map to the quantum domain.
There are several reasons for that. First, today’s computer algorithm tries to optimise the parameters using methods such as gradient descent, but quantum measurements are noisy. Plus, quantum programs don’t support tons of parameters of traditional computers.
As a result, the scientists are developing QML programs to take advantage of the unique properties of QC technology while sidestepping the limitations of QC systems. With all this, the prospects of QML appear daunting yet fascinating and promising.